Abstract
The research aims to identify the function of big data analytics to attain competitive advantage through the mediation of business model innovation.260 samples were taken from experts in Pakistan's manufacturing & service sectors. Data analysis was done using (Smart PLS). The findings demonstrate that big data analysis capability (BDAC) has a considerable impact on competitive advantage. By using big data, companies can make rational, data-driven decisions to satisfy customers, sustain and resilient supply chain management, low production costs, and efficient and effective business operations. Similar to how BC affects competitive advantage, business model innovation partially mediates this effect. Additionally, the influence of BDAC on both competitive advantages & new business model innovation is moderated by organizational IT infrastructure. This research presents a unique scenario of big data analytics in which firms use bid data inside to innovate their established business models, resulting in considerable competitive advantage in emerging countries like Pakistan.
Key Words
: Big Data, Competitive Advantage, Innovation, Dynamic Capability
Introduction
Organizations in the current scenario of a more complex and uncertain business environment must engage in innovation; recreating and modifying their strategic capabilities to counter this environment from a dynamic capability theoretical view (Al-Darras & Tanova, 2022; Lerman et al., 2022; Ramadan et al., 2020). Only making capital investments will not be enough to compete; firms should have to create innovative capabilities that competing firms will find difficult to imitate (Dubey et al., 2018; Lerman et al., 2022; Shamim et al., 2019). As a direct result of the digital revolution, society is undergoing profound and rapid transformation. As a consequence of this, companies are looking for innovative methods to entice new customers, satisfy existing ones, and retain existing ones. The firms can attempt to gain a competitive advantage by adopting innovative strategies (Shah, 2022) Organizations are using digital technologies such as big data techniques to create innovation in their businesses (Kubina et al., 2015; Manyika & Roxburgh, 2011; Wiener et al., 2020). It has the potential to enhance firms' competitive advantages by improving data-driven performance (Ciampi et al., 2021a; Ramadan et al., 2020).
A firm's underlying framework may be described as its "business model." (Naomi et al., 2016); a description of the strategies that your organization plans to use to generate value in the market (Geissdoerfer et al., 2018) It comprises the underlying organization of people as well as the operational infrastructure that individuals use to get their tasks done (Ciampi et al., 2021a; Rachinger et al., 2019). Innovating business models has become essential for survival in the competitive world (Garzella et al., 2021). Business models are made to fulfil consumers' needs. All strategies of the business are kept aligned with the business model (Spieth et al., 2019; Veile et al., 2022).
Big data enables the provision of useful information to execute fully data-based business models (Ghasemaghaei & Calic, 2019). Modern data analytics tools can identify the root causes of production system variance and develop countermeasures to increase system stability and save costs.(Cochran et al., 2016) Firms can effectively foresee market requirements by using BDA approaches; as a result, they can innovate and adapt their business model strategies (Gupta et al., 2020). The business environment is rapidly changing; therefore, it is difficult to sustain innovative ideas without data analysis(Bocken et al., 2014; Geissdoerfer et al., 2018). Competitive advantage is crucial for the success of strategic decisions and management (Dahiya et al., 2022; Sigalas, 2015). By using big data and innovative business techniques, a competitive advantage can be gained (Ciampi et al., 2021b; Dahiya et al., 2022).
This study examines the relationships between big data analytics, business model innovation, and competitive advantage under the moderating effect of organizational IT infrastructure. In today's database knowledge-intensive economy, information technology (IT) is one component that cannot be overlooked. Without effective information technology, effective big data management is not conceivable (Saeidi et al., 2019). Organizational IT infrastructure is an essential building block for firms that seek to maximize the potential of emerging digital technologies (Klotins & Peretz-Andersson, 2022). Firm processes, networks, and organizational and technical components establish an IT infrastructure (Joglekar et al., 2022). IT infrastructure is identified in the research literature as the linkage of various system collections such as software, hardware, standard procedures, the Internet, and very important people (Hustad & Olsen, 2021). To facilitate the integration of their internal business processes, firms need IT strategies and procedures that may increase productivity, become more efficient, and react quickly to customer needs (Jha et al., 2020). To successfully achieve and sustain competitive advantage, firms must develop a proper IT infrastructure, which lays a technological foundation for innovation and competitiveness through advanced data analytic capabilities like big data. The ability of an organization to adapt to change is a crucial aspect in the development of big data analytics capabilities. It can be impossible to build big data analytics if the current IT infrastructure is wholly incompatible with it, as managers are not able to make data-driven decisions, and are also unable to operate the systems (Rialti et al., 2019). Companies are using bid data in the decision-making process (Gupta et al., 2021). Companies spend their significant budgets on IT, digitalization, and data analytics (Grover et al., 2018). Any amount of data is worthless if you don't have proper infrastructure and people who know how to make use of it (Wicaksana, 2016). So big data analytics as a resource capability provides a common pool of information technology (IT) resources for innovation, which is the most critical criterion in achieving competitive advantage.
The business process is increasingly changing due to the prevalence of digitalization, advancement in information technology, the Internet of Things, and big data analytics. Therefore, the adoption of these elements is essential, along with a refined and innovative business model. (Parida et al., 2019). The IT infrastructure of an organization strategically affects its capability to utilize advanced data analytics to innovate its business models (Ciampi et al., 2021b; Rehman et al., 2020). Even though big data analytics (BDA) advancements offer tremendous commercial value and useful business insights, many organizations find it challenging to benefit from their BDA endeavours (Dahiya et al., 2022). However, many organizations are still not utilizing the full potential of big data analytics as a resource capability (Tabesh et al., 2019).
To fill this gap, we create a framework to examine the relationship between big data analytics as an independent variable and competitive advantage as a dependent variable while taking into consideration the strategic role of business model innovation as a mediator. Additionally, we also examine the moderating effect of a firm’s IT infrastructure with BDA on business model innovation and competitive advantage separately. A huge volume of data termed Big Data, was collected in organizations, but unable to analyze and interpret the data for strategic decision-making in this competitive market, which is of great concern for the industrial sector (Awan et al., 2022). The subject of how firms may improve their competitive advantage by utilizing big data analytics capability with the mediating role of business model innovation has not been addressed by the current literature, although it has identified the factors that affect the competitive advantage and firm performance through big data (Al-Darras & Tanova, 2022). This study aims to provide an answer to the question "How can big data analytical enhance competitive advantage, and how can business model innovation contribute to competitive advantage?" based on the discussion above.
Literature Review
Big Data Analytics
There is great importance to researching big data analytics (Dubey et al., 2019). Information flows within and across organizations have become crucial for the survival of business growth (Ciampi et al., 2021b). Big data and predictive analytics capability are regarded as two of the most important organizational resources in the age of digital transformation (Al-Darras & Tanova, 2022). If organizations can explore value by utilizing the potential of big data, big data may be an extremely useful resource capability for them The development of technologies like big data analytics is providing new horizons for firms to give value to their consumers in terms of cost-effectiveness (Åström et al., 2022; Toufaily et al., 2021). Dynamic capabilities, or DCs, are practical, simple, and flexible procedures that depend on quickly developed new insights to facilitate the transformation, combination, or renewal of resources and competencies into capabilities that are required for ambiguous markets in a highly uncertain world (Eckstein et al., 2015; Eisenhardt and Martin, 2000). According to the above ideas, BDA is viewed as a dynamic capability that arises from an organization's ability to reconfigure firm-level resources (Wamba & Ren, 2017).
Competitive Advantage
Porter (1985) claims that corporations can achieve a competitive advantage by finding and implementing generic strategies, and he discusses the interaction between several types of competitive advantage—cost and distinctiveness—and the breadth of a firm's activities. "A corporation is said to have a competitive advantage when it is implementing a value-creating strategy that is not being executed by any present or potential competitors," according to Barney (1991, p. 102). According to Peteraf (1993), the capacity of an organization to maintain above-average returns is referred to as a competitive advantage. According to Reed and DeFillippi (1990), competitive advantage can be used for various purposes (Hinterhuber, 2013).
Business Model Innovation
A company's "business model" is essentially a summary of its "business structure." (Baden-Fuller & Morgan, 2010). The literature has highlighted three key BM dimensions: value creation, value proposition, and value capture (Clauss, 2017). BMI may be described as the process of consciously changing one or more components that are fundamental to the business value logic for the benefit of the organization, its clients, and any other relevant stakeholders. (Bucherer et al., 2012). It is necessary to take full advantage of disruptive technologies since developing innovative technological solutions is just as important as coming up with new business models
(Åström et al., 2022). A business model offers the framework for how an enterprise grows and delivers value to customers, as well as the strategies employed to gain a component of that value.
(Rachinger et al., 2019). Business model innovation (also known as BMI) is the primary strategy for overcoming external difficulties through the process of developing the foundation of a company. (Kraus et al., 2022).
Big Data Analytics and Competitive Advantages
BDA has been recognized as a viable instrument for strengthening firms' competitive advantage by improving data-driven performance (Ramadan et al., 2020). Big data analytics, when used as a dynamic capability, can be used to generate knowledge that is relevant to business, provide value, improve performance, and give a firm an advantage over rivals in a dynamic market (Singh & Del Giudice, 2019). Firms that successfully utilize the digitalization potential of IT infrastructure powered by big data and analytics will outperform their rivals in terms of revenue growth and operational effectiveness(Cappa et al., 2021; Parida et al., 2019). According to Chen et al. (2012), big data presents a huge possibility for gaining a competitive advantage. BDA results that include custom applications and firm internal data will provide high-level firm-specific knowledge and could lead to a long-term competitive advantage (Dahiya et al., 2021). This discussion leads to the hypothesis:
H1: BDA has a positive effect on Competitive Advantage
Big Data Analytics, Business Model Innovation, and Competitive Advantage
The availability of BDA, in particular, allows companies to tap into the value of useful insights and information generated from diverse, large-scale, and up-to-date information in the form of data about consumers, competitors, and markets (Ghasemaghaei et al., 2019) to fully implement and apply data-driven business models (Manyika et al., 2011). Firms may accurately foresee market requirements and, as a result, change their strategies and structures to satisfy evolving markets' wants and reveal upcoming market goals by employing BDA approaches (Gupta et al., 2019). The key benefit of data-driven BMI is the capacity to rationalize management's intuitions and inventiveness by providing rapid and ongoing access to new information about business stakeholders (Cheah & Wang, 2017).
BMI is found to be a strong predictor of competitive advantage (Porter's competitive strategy). Because rivals promptly imitate a company's strategy if it suffers from BMI, businesses must frequently alter their BM to gain a stronger competitive advantage. (Mitchell and Coles, 2003). In contrast to SMEs with reactive BMs, those with updated and proactive BMIs increase their value and attract more customers in dynamic marketplaces. (Hacklin et al., 2017; Shahzad et al., 2017). The main forces behind the company's sustainability and values are cost reduction, risk reduction, reputation, brand image, attractiveness for employees, and new skills that BMI can cover. (Schaltegger et al., 2012).
This discussion leads to the hypothesis
H2: BMI positively mediates between BDA and Competitive Advantage
The moderating effect of Organizational IT Infrastructure
The importance of information
technology (IT) in today's economic and commercial environment cannot be
denied. Business firms can now use IT for marketing, sharing information on many platforms, data
transformation, monitoring, quality control, e-commerce, etc. (Massachusetts, 2002). In addition, IT is
considered a tool to increase competitive advantage (Mao et al., 2016; Marinagi
et al., 2014). If a company learns that
its competitors are employing digital technology like big data, it will
experience a crisis as it realizes that without big data analytics, it will
soon lose its competitive advantage (Chen et al., 2015). Furthermore, past
research has shown that the stronger a company's IT capabilities are, the more
likely it is to adopt new technologies (Kuan and Chau, 2001; Kamal, 2006). As a
result, it is thought that a company's IT infrastructure and competencies can boost the likelihood
of BDA adoption. The above discussion leads to the following hypotheses for
testing:
H3: Organizational
IT infrastructure moderates the effect of BDA on Competitive Advantage
Increased digitalization and sophisticated IT infrastructure
have had an impact on business models because they have allowed companies to
collaborate in new ways, leading to the development of new products and
services as well as new channels for communication with clients and staff. (Rachinger et al., 2019). Simultaneously,
increasing digitization has increased pressure on companies to assess their
current approach and extensively research new business opportunities (Bouwman et al., 2019; Rachinger et al., 2019).
The following hypothesis is derived from the above
discussion:
H4: Organizational
IT infrastructure moderates the effect of BDA on BMI
Theoretical underpinning
The dynamic capability theory (DCT) is used to describe the underlying relationship between the variables in this research. It highlights the effect of BDA on a firm's competitive advantage through the mediating role of BMI. The Dynamic Capability (DCT) theory originated as a theoretical addition and extension of the resource-based view (RBV) theory to describe how organizations manage to stay competitive. in unpredictable circumstances throughout time (Ambrosini & Bowman, 2009). According to the fundamental idea of the resource-based view theory, a company's success is determined by the accessibility and organization of unique, inimitable, non-substitutable, and valuable assets, which permit the adoption of value-creating strategies for a firm (Barney, 1991). A corporate firm can achieve a long-term competitive advantage by obtaining and controlling strategic resources and, as a result, establishing firm-specific skills that are extremely dependent on the types of resources gathered (Makadok, 2001). In recent times, research has shown that the RBV's static. The approach falls short of understanding how firms use their resources and capabilities in dynamic marketplaces, opening the door for the DCV's widespread adoption (Priem & Butler, 2001). The dynamic capabilities theory (DCT) suggests that firms should be skilled in recreating and renewing their competitive strategies and capabilities to be in a competitive position to meet the challenges of a dynamic and uncertain environment (D.J. Teece et al., 1997; G. Linden et al., 2018). DCs enable firms to build unique organizational and strategic routines and skills that are critical to their continued success and the creation of new markets (Fornell & Larcker, 1981).
Figure 1
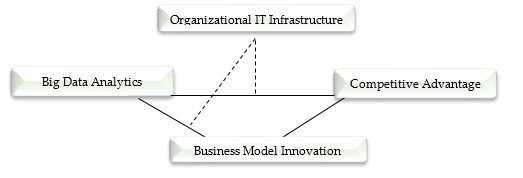
The dynamic capability and resource-based theory provide the theoretical underpinnings of the above theoretical framework. The relationship depicted in Model IV, DV, has been taken from the previous literature, as mentioned above. However, the mediating and moderating variables shown in Figure 1 have been tested separately for all variables. However, the current model is unique as the relationship between mediating and moderator has not been tested before in the Pakistani context. This unique model has been developed by the author.
Methodology
This study's methodology was precisely developed to ensure rigour. The research philosophy used in this study is positivism with a cross-sectional survey-based and quantitative design (Alharahsheh et al., 2020). This research used a deductive approach, relying on theoretical explanations based on observable events and hypothesis testing. This strategy is used to establish a causal link between variables, test hypotheses, and generalize the regularities of human social behaviour (Saunders et al., 2007). To support us in data collection, we contacted professionals in the manufacturing and service industries with field experience of at least 1 year, working in both multi-national and national firms. The manufacturing sector contributes 19.3% and the service sector contributes 58 % to the GDP of Pakistan (Pakistan Economic Survey, 2022) In today's world, both the service and industrial industries are being afflicted by a digital tsunami. (Zhong et al., 2016) The questionnaire consisted of 33 items, and to access our theoretical model, 268 samples were gathered all over Pakistan's manufacturing and service industries in November and December 2023. Structural Equation Modeling (PLS-SEM) was chosen as the statistical analysis method since it has both explanatory and predictive power and can be used for non-normal data distribution.
Measures
The survey questionnaire is based on 4 parts; BDA, organization IT infrastructure, BMI competitive pressure, competitive advantage, and type of industry. BDA, which is a study of the second-order independent variable and its construct items, was adopted and assessed (Dubey et al., 2018). Each item of BMI, which is a study mediating variable, was adopted and assessed (Ciampi et al., 2021b). A competitive advantage, which is a study-dependent variable, and its construct items were adopted and assessed (Abeysekara et al., 2019). Each item of the organization's IT infrastructure, which is a study moderating variable, is evaluated and assessed (Yuanyuan et al., 2017). All items of the above-mentioned variables were evaluated by respondents on a Likert scale from 1 (strongly disagree) to 5 (strongly agree). Industry type, which is a categorical variable, is taken as a control variable in this study.
Results
The results and discussion chapter presents in detail the results from the data analysis. PLS-SEM analysis contains measurement and a structural model. The measurement model analyzes the reliability and validity of the constructs. The relevance of proposed linkages is ascertained using the structural model.
Sample Description and Demographics
The information was gathered between 01 Nov 2023 and 05 Dec 2023. The questionnaire was floated through Google Forms to 350 people. Out of 268 responses received, 8 were not filled out properly, therefore 260 responses were analyzed. Details are given below:
Table 1
Frequency
Percentage (age)
Designation
Staff Level Manager
12
4.5
Lower Level Manager
66
24.7
Middle-Level Manager
95
35.6
Senior Level Manager
77
28.8
Top/ Executive Level
17
6.4
Total
267
100
Job Experience
1-5 years
106
39.8
6-10 years
56
21.1
11-15 years
40
15.0
16-20 years
45
16.9
Above 20 years
19
7.1
Total
266
100
Education
Matriculation
01
0.7
Intermediate
01
0.7
Graduate
110
41.0
Masters
156
58.2
Total
268
100.0
Common Method Bias (CMB)
Harman's single-factor analysis revealed that there was no common method bias variance because the highest-ranking factor only explained 66.1 % of the variation (Podsakoff et al., 2003). CMB was next evaluated and ruled out since the latent variables' factor level variance inflation factor (VIF) was likewise less than 3.3 (Kock, 2015), as shown in Table 3.
Table 2
Construct
VIF
BDA
2.081
BM
2.292
CA
2.473
Measurement Model
According to Sarstedt et al. (2019), researchers have proposed the two-stage approach as an alternative to the repeated indicators approach. In the context of PLS-SEM, higher-order constructs (also known as hierarchical component models) provide a framework for researchers to model a construct on a more abstract dimension (called a higher-order construct) and its more concrete sub-dimensions (referred to as lower-order constructs).
Second-Order Reflective Measurement Model
Create and estimate the model that connects all of the lower-order components in the first step (including exogenous and endogenous constructs). The reflecting measurement models of the lower-order components are the focus of the model evaluation initially. In stage two, you develop and estimate the stage two models using the latent variable scores of the lower-order components from stage one. Locate the higher-order constructs of the lower-order constructs' scores and add them as new variables to the dataset for this purpose. The results are comparable to those obtained using the repeated indicators approach, with the path coefficient estimates differing somewhat. The second stage's evaluation begins with a focus on the higher-order component's reflective measurement model. See the lower-order construct loadings for the higher-order construct, then use the coefficients (loadings) to analyze the composite reliability and average value extracted, allowing us to build indicator reliabilities and AVE. These results establish the reliability and convergent validity above the crucial value of 0.5 Cronbach's alpha, as well as the composite reliability, and average value extracted.
The reliability and validity of the item and constructs are calculated by testing the measurement model. The composite reliability, convergent validity, and discriminant validity are above the prescribed threshold values (Joseph F. Hair et al., 2019). Detail has been given in Table 3 and Table 4 below
Table 3
Constructs
Items
Outer Loadings
Composite Reliability
Average Variance Extracted (AVE)
Business Model Innovation
BM1
0.773
0.914
0.681
BM2
0.849
BM3
0.864
BM4
0.789
BM5
0.847
Competitive Advantage
CA1
0.804
0.904
0.612
CA2
0.835
CA3
0.796
CA4
0.792
CA5
0.772
CA6
0.686
DDDMC
DC1
0.753
0.869
0.571
DC2
0.835
DC3
0.727
DC4
0.702
DC5
0.755
Managerial Skills
MS1
0.792
0.914
0.640
MS2
0.801
MS3
0.766
MS4
0.821
MS5
0.834
MS6
0.784
Discriminant validity is established based on the hetero-trait–monotrait ratios. For each construct, the HTMT ratio is less than 0.90 (Henseler et al., 2015). HTMT ratio is based on the estimation of the correlation of the construct. The HTMT ratio results in Table 4 below show that all HTMT ratios are less than the required threshold of 0.9 except the BDA construct.
Table 4
BDA
BM
CA
DC
MS
TS
BDA
0.00
0.00
0.00
0.00
0.00
0.00
BM
0.649
0.00
0.00
0.00
0.00
0.00
CA
0.57
0.703
0.00
0.00
0.00
0.00
DC
0.964
0.664
0.699
0.00
0.00
0.00
MS
1.003
0.596
0.456
0.773
0.00
0.00
TS
0.998
0.552
0.464
0.745
0.837
0.00
Abbreviations: BDA; Big Data Analytics, BM; Business Model Innovation
CA; Competitive Advantage, DC; Data Driven Decision Making Culture
MS; Managerial Skills , TS; Technical Skills
Multicollinearity between constructs was checked through collinearity statistics and VIF values in PLS-SEM. A previous study revealed that for a reasonable degree of multicollinearity, the variance inflation factor (VIF) must be less than 3.3 (Hair et al., 2016). We found that all VIF values were less than 3.3, indicating that there are no difficulties with collinearity.
Table 5
BDA
BM
CA
DC
MS
TS
BDA
0.00
1.00
1.533
1.00
1.00
1.00
BM
0.00
0.00
1.533
0.00
0.00
0.00
CA
0.00
0.00
0.00
0.00
0.00
0.00
DC
0.00
0.00
0.00
0.00
0.00
0.00
MS
0.00
0.00
0.00
0.00
0.00
0.00
TS
0.00
0.00
0.00
0.00
0.00
0.00
Figure 2
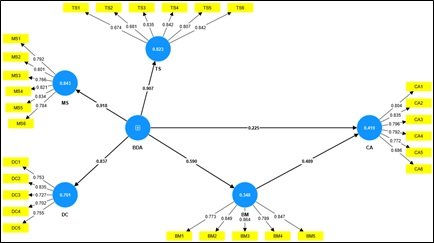
Second-Order Reflective-Measurement Model
As part of the measurement model validation, the second or highest-order constructs were also validated. Each of these constructs was evaluated for reliability and convergent validity. Sarstedt et al. (2019) proposed that the higher-order construct be examined for discriminant validity with other lower-order constructs in the research. According to the results, the reliability and validity of the higher-order constructs have been established. For all other constructs, reliability and convergent validity are established when the reliability value is greater than 0.70 and the AVE is greater than 0.50, respectively (Table 5). Discriminant validity of the higher-order construct with lower-order constructs is also tested in addition to reliability and validity.
Table 6
Construct
Items
Outer Loadings
Composite Reliability
Average Variance Extracted (AVE)
BDA
DC
0.883
0.918
0.788
MS
0.894
TS
0.885
Variance Explained (Convergent Validity)
Discriminant validity with other lower-order constructs is established using the HTMT criterion, which is less than 0.90 (Henseler et al., 2015) as shown in Table 6 below.
Variance Explained (Convergent Validity)
Discriminant validity with other lower-order constructs is established using the HTMT criterion, which is less than 0.90 (Henseler et al., 2015) as shown in Table 6 below.
Table 7
BDA
BM
CA
BDA
0.00
0.00
0.00
BM
0.678
0.00
0.00
CA
0.606
0.703
0.00
BDA
DC
MS
TS
BDA
0.720
0.766
DC
0.836
MS
0.929
0.651
0.825
TS
0.920
0.673
0.608
0.868
Figure 3 (a)
.jpg)
Figure 3 (b)
.jpg)
Structural-Model Measurement
The evaluation of the structural model is the subsequent phase in the evaluation process, as depicted in Figure 2 above. In order to assess the statistical significance of the PLS-SEM results, bootstrapping with 5000 iterations was utilised for the development of the structural model. These included the structural path coefficient (beta), R2, Q2, and f2 values. The prediction ability and model fit were assessed using R2 and Q2 values. The coefficient of determination R2 was employed for each endogenous variable in the model to assess the quality of the generated structural equation model (SEM). The coefficient of determination (R2) for the dependent variable BMI is 0.564, whereas for the dependent variable competitive advantage, it is 0.536, as indicated in Table 7. The R2 value exceeds 0.2, indicating that the model possesses an adequate level of explanatory capability for the fluctuations observed in the dependent variables (Hair et al., 2016). The data presented in Table 7 clearly demonstrates that both BDA and BMI account for a significant 53.6.1% of the variability seen in competitive advantage. The BDA accounted for 56.4% of the variability in BMI, indicating a significant relationship.
In addition to the goodness-of-fit measurements, the effect size coefficients (f2) were utilised to evaluate the suitability of the developed model in Figure 2. Based on the findings of Hair et al. (2017), the effect size f2 values of 0.02, 0.15, and 0.35 represent minor, medium, and large effects, respectively, for endogenous latent variables. These values are reported in Table 7. All other f2 values above the range of 0.02, indicating acceptable levels of impact size. Furthermore, the blindfolding technique was utilised to assess the model's anticipated accuracy (Akter et al., 2011). The route model's predictive significance is satisfied if the Q2 value for a reflecting endogenous variable is greater than zero (Sarstedt et al., 2017). Table 7 demonstrates that the predictive relevance of the constructs was over zero, which is within the allowed range for all endogenous components, hence establishing predictive relevance.
Table 8
Total effect size (f2)
coefficient of determination (R2)
Predictive relevance (Q2)
BMI
CA
BDA
0.105
0.065
-
-
BMI
-
0.267
0.564
0.397
CA
-
0.536
0.251
The next step is structural-equation modelling, which is an assessment of the hypothesized relationship to substantiate the proposed hypotheses.
Direct Effects
To test the hypotheses, the inner model is evaluated using boot-strapping with 5000 subsamples. The hypothesized direct impact path-coefficients, T values, effect size, and supported or not decision are summarized in Table 9. H1 evaluates whether BDA has a significant impact on the competitive advantage or not. The results revealed that BDA has a significant effect on competitive advantage (? =0.265, T=2.851, p = 0.002). Hence, H1 is supported in this case. The exogenous variable's contribution to R2 is represented by the effect size f2; the value of 0.02 is considered a small-size effect, 0.15 is considered a medium-size effect, and 0.35 is considered a large-size effect (Hair et al., 2016). In this model, all supported hypotheses have a small but statistically significant impact size.
Mediation (Indirect) Effect
One simple mediation path is included in the proposed model. The result shows that the mediation's indirect effect is supported. A mediation analysis was performed to assess the mediating role of BMI. BMI mediates between BDA and competitive advantage. The results in Table 9 below, revealed a significant (p =0.000 and ? =0.171) partial mediating role of BMI.
Moderation Effects
The moderation results in Table 10 confirm the hypothesis (H3) that IT infrastructure moderates the effect of BDA (p=0.021, ? =0.421) on competitive advantage, but on the other side firm IT infrastructure ((H4), also moderates (p=0.019, ? =0.161) the effect of BDA on business model innovation BMI as shown in table 9.
Table 9
Direct Effects, Indirect Effects, and Moderating Effects
Relationships Beta coefficient Std Error T-Statistics Confidence Interval P-value Decision
5% 95%
Directs Effect
BDAà CA 0.265 0.093 2.851 0.117 0.423 0.002 Supported
Indirect Effect
(Mediation)
BDAàBMI àCA 0.171 0.044 3.905 0.102 0.246 0.000 Supported
Moderation Effects
BDA*IT à CA 0.421 0.047 2.579 0.074 0.166 0.021 Supported
BDA*IT à BMI 0.161 0.077 2.077 0.149 0.018 0.019 Supported
Importance Performance Map Analysis (IPMA)
The importance-performance map analysis is an innovative method for presenting PLS-SEM results in Smart PLS version 3. It is commonly employed to evaluate the effectiveness of crucial aspects that contribute to the success of a firm. The x-axis represents the "importance" or overall impact of business success drivers, measured on a scale from 0 to 1. The y-axis represents the "performance" of these drivers, measured on a scale from 0 to 100. Subsequently, researchers can utilise this data to pinpoint past structures that have a substantial overall impact (high Importance) but exhibit low average latent variable scores (bad performance). This knowledge can be applied to enhance operational efficiency in the future (Joe F Hair et al., 2018). Figure 3 illustrates that in this model, Organisational IT infrastructure holds greater significance and exhibits higher performance compared to BDA (Big Data Analytics) and BMI (Business Model Innovation). Managers should not underestimate the importance of this area in order to achieve a lasting competitive advantage. The combination of big data analytics and business model innovation has a significant impact on both importance and performance, playing a crucial role in achieving a competitive advantage.
Figure 4
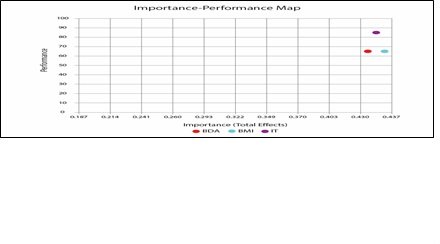
PLS-Predict
When it comes to predicting model parameters for new observations, explanatory modelling is not very reliable (Hair & Sarstedt, 2021). On the other hand, predictive power refers to a model's ability to make reliable predictions for new observations (Shmueli & Koppius, 2011). The model was tested to see if it could have predictive relevance. As shown in Table 9, for all dependent variables, PLS-SEM Q2 predicts one is larger than zero except for four indicators. All of the PLS-SEM indicators had a lower RMSE (prediction error statistic) than the linear model. As a result, the model's medium predictive potential is determined (Shmueli et al., 2019) through this analysis.
Table 10
PLS-SEM
LM
PLS-SEM - LM
Item
RMSE
Q²predict
RMSE
RMSE
BM3
0.878
0.266
0.898
-0.02
BM2
0.835
0.262
0.85
-0.015
BM4
0.801
0.311
0.812
-0.011
BM1
0.91
0.212
0.911
-0.001
BM5
0.83
0.291
0.846
-0.016
CA2
0.866
0.182
0.851
0.015
CA5
0.818
0.159
0.784
0.034
CA4
0.949
0.095
0.92
0.029
CA3
0.875
0.156
0.876
-0.001
CA6
0.88
0.168
0.871
0.009
CA1
0.926
0.158
0.927
-0.001
Discussion
Overall, in this study, we demonstrated how big data analytics capability, business model innovation, and organizational IT infrastructure can be effectively utilized in advanced data analytics and innovation for the attainment of competitive advantage. Having the firm resources to compete is not so essential but effective utilization of these resources is much more important to generate significant value from those resources (Barlette & Baillette, 2022). Particularly in a dynamic and uncertain environment, firms need to reconfigure their present capabilities; that is the key argument of dynamic capabilities theory (Shamim et al., 2019). This study examined how dynamic capabilities development can be influenced by big data analytics, business model innovation, and organizational IT infrastructure that leads toward sustainable competitive advantage.
The findings of our study corroborate the findings of earlier research by showing a favourable and substantial association between big data analytics and competitive advantage (Dahiya et al., 2022; Ramadan et al., 2020). Furthermore, the relationship between BDA and CA is strengthened by the moderating function of IT infrastructure and capabilities, which also offers the technological basis for this fruitful partnership.
There is potential for businesses to leverage big data analytics as a resource capability, according to theoretical literature about the relationship between big data analytics and business model innovation (Ciampi et al., 2021b; Rachinger et al., 2019). Furthermore, organizations may benefit from the theoretical literature's examination of the connection between business model innovation and competitive advantage (Anwar, 2018; Azeem et al., 2021; Ramadan et al., 2020). Nevertheless, in empirically based research, the moderating function of IT infrastructure with BDA on BMI and CA and the mediating role of business model innovation between BDA and CA remain to be investigated. Big data analytics and competitive performance have been found to be directly and favourably correlated by this study, which supports the theoretical perspective of dynamic capabilities theory, which views big data analytics as a resource capability in an unpredictable and dynamic business environment (Ramadan et al., 2020).
Conclusion
The enormous interest that practitioners and scholars have shown in the big data phenomena served as a major driving force behind our study. This research work adds to the growing body of empirical research on the value of strategic and innovation management's ability to effectively utilize business development practices in the current scenario of the uncertain, complex, highly competitive, and dynamic environment of doing business. By making use of big data, businesses can make choices that are rationally based on data to satisfy consumers, maintain and strengthen supply chain management, maintain low production costs, and ensure efficient and successful corporate operations in the manufacturing and service sector of Pakistan.
It also demonstrates how business firms' efforts to create adaptable technological frameworks and innovative big data analysis capabilities support a corporate data-driven culture that can facilitate the adoption of various business model innovation processes. Moreover, it reveals that business model innovation has a positive and partial mediation role in the relationship between big data analytics and competitive advantage, indicating that the availability of sufficient big data resources and capabilities encourages the adoption of a strategic business model innovation inclination characterized by high levels of creativity, resiliency, and risk-taking, which, in turn, makes it easier to compete successfully in the market of the more dynamic, complex and highly competitive business environment.
References
- Abeysekara, N., Wang, H., & Kuruppuarachchi, D. (2019). Effect of supply-chain resilience on firm performance and competitive advantage. Business Process Management Journal, 25(7), 1673–1695. //doi.org/10.1108/bpmj-09-2018-0241
- Al-Darras, O. M. A., & Tanova, C. (2022). From Big Data Analytics to Organizational Agility: What Is the Mechanism? SAGE Open, 12(2). https://doi.org/10.1177/21582440221106170
- Alharahsheh, H. H., & Pius, A. (2020). A Review of key paradigms: positivism VS interpretivism. Global Academic Journal of Humanities and Social Sciences, 2(3), 39–43. https://www.researchgate.net/publication/338244145
- Ambrosini, V., & Bowman, C. (2009). What are dynamic capabilities and are they a useful construct in strategic management? International Journal of Management Reviews, 11(1), 29–49. https://doi.org/10.1111/j.1468-2370.2008.00251.x
- Anwar, M. (2018). Business model innovation and SMEs performance-Does competitive advantage mediate? International Journal of Innovation Management, 22(7), 1–31. https://doi.org/10.1142/S1363919618500573
- Åström, J., Reim, W., & Parida, V. (2022). Value creation and value capture for AI business model innovation: a three-phase process framework. Review of Managerial Science, 16(7), 2111–2133. https://doi.org/10.1007/s11846-022-00521-z
- Awan, U., Bhatti, S. H., Shamim, S., Khan, Z., Akhtar, P., & Balta, M. E. (2022). The Role of Big Data Analytics in Manufacturing Agility and Performance: Moderation– Mediation Analysis of Organizational Creativity and of the Involvement of Customers as Data Analysts. British Journal of Management, 33(3), 1200–1220. https://doi.org/10.1111/1467-8551.12549
- Azeem, M., Ahmed, M., Haider, S., & Sajjad, M. (2021). Expanding competitive advantage through organizational culture, knowledge sharing and organizational innovation. Technology in Society, 66(June), 101635. https://doi.org/10.1016/j.techsoc.2021.101635
- Barlette, Y., & Baillette, P. (2022). Big data analytics in turbulent contexts: towards organizational change for enhanced agility. Production Planning and Control, 33(2–3), 105–122. https://doi.org/10.1080/09537287.2020.1810755
- Bocken, N. M. P., Short, S. W., Rana, P., & Evans, S. (2014). A literature and practice review to develop sustainable business model archetypes. Journal of Cleaner Production, 65, 42–56. https://doi.org/10.1016/j.jclepro.2013.11.039
- Bouwman, H., Nikou, S., & de Reuver, M. (2019). Digitalization, business models, and SMEs: How do business model innovation practices improve performance of digitalizing SMEs? Telecommunications Policy, 43(9), 101828. https://doi.org/10.1016/j.telpol.2019.101828
- Cappa, F., Oriani, R., Peruffo, E., & McCarthy, I. (2021). Big Data for Creating and Capturing Value in the Digitalized Environment: Unpacking the Effects of Volume, Variety, and Veracity on Firm Performance*. Journal of Product Innovation Management, 38(1), 49– 67. https://doi.org/10.1111/jpim.12545
- Chen, D. Q., Preston, D. S., & Swink, M. (2015). How the use of big data analytics affects value creation in supply chain management. Journal of Management Information Systems, 32(4), 4–39. https://doi.org/10.1080/07421222.2015.1138364
- Ciampi, F., Demi, S., Magrini, A., Marzi, G., & Papa, A. (2021a). Exploring the impact of big data analytics capabilities on business model innovation : The mediating role of entrepreneurial orientation. Journal of Business Research, 123(June 2020), 1–13. https://doi.org/10.1016/j.jbusres.2020.09.023
- Ciampi, F., Demi, S., Magrini, A., Marzi, G., & Papa, A. (2021b). Exploring the impact of big data analytics capabilities on business model innovation: The mediating role of entrepreneurial orientation. Journal of Business Research, 123(September 2020), 1– 13. https://doi.org/10.1016/j.jbusres.2020.09.023
- Cochran, D. S., Kinard, D., & Bi, Z. (2016). Manufacturing System Design meets Big Data Analytics for Continuous Improvement. Procedia CIRP, 50, 647–652. https://doi.org/10.1016/j.procir.2016.05.004
- Dahiya, R., Le, S., Ring, J. K., & Watson, K. (2022). Big data analytics and competitive advantage: the strategic role of firm-specific knowledge. Journal of Strategy and Management, 15(2), 175–193. https://doi.org/10.1108/JSMA-08-2020-0203
- Dubey, R., Gunasekaran, A., Childe, S. J., Blome, C., & Papadopoulos, T. (2019). Big Data and Predictive Analytics and Manufacturing Performance: Integrating Institutional Theory, Resource-Based View and Big Data Culture. British Journal of Management, 30(2), 341–361. https://doi.org/10.1111/1467-8551.12355
- Dubey, R., Gunasekaran, A., Childe, S. J., Luo, Z., Wamba, S. F., Roubaud, D., & Foropon, C. (2018). Examining the role of big data and predictive analytics on collaborative performance in context to sustainable consumption and production behaviour. Journal of Cleaner Production, 196, 1508–1521. https://doi.org/10.1016/j.jclepro.2018.06.097
- Garzella, S., Fiorentino, R., Caputo, A., & Lardo, A. (2021). Business model innovation in SMEs: the role of boundaries in the digital era. Technology Analysis and Strategic Management, 33(1), 31–43. https://doi.org/10.1080/09537325.2020.1787374
- Geissdoerfer, M., Vladimirova, D., & Evans, S. (2018). Sustainable business model innovation : A review. Journal of Cleaner Production, 198, 401–416. https://doi.org/10.1016/j.jclepro.2018.06.240
- Ghasemaghaei, M., & Calic, G. (2019). Does big data enhance firm innovation competency? The mediating role of data-driven insights. Journal of Business Research, 104(July), 69–84. https://doi.org/10.1016/j.jbusres.2019.07.006
- Grover, V., Chiang, R. H. L., Liang, T. P., & Zhang, D. (2018). Creating Strategic Business Value from Big Data Analytics: A Research Framework. Journal of Management Information Systems, 35(2), 388–423. https://doi.org/10.1080/07421222.2018.1451951
- Gupta, S., Drave, V. A., Dwivedi, Y. K., Baabdullah, A. M., & Ismagilova, E. (2020). Achieving superior organizational performance via big data predictive analytics: A dynamic capability view. Industrial Marketing Management, 90, 581– 592. https://doi.org/10.1016/j.indmarman.2019.11.009
- Gupta, S., Justy, T., Kamboj, S., Kumar, A., & Kristoffersen, E. (2021). Big data and firm marketing performance: Findings from knowledge-based view. Technological Forecasting and Social Change, 171(1). https://doi.org/10.1016/j.techfore.2021.120986
- Hair, J. F., Risher, J. J., Sarstedt, M., & Ringle, C. M. (2019). When to use and how to report the results of PLS-SEM. European Business Review, 31(1), 2–24. https://doi.org/10.1108/ebr-11-2018-0203
- Hair, J. F. (2021). Reflections on SEM: An Introspective, Idiosyncratic Journey to Composite-Based Structural Equation Modeling. The DATA BASE for Advances in Information Systems, 52(SI), 101–113. http://dx.doi.org/10.1145/3505639.3505646
- Henseler, J., Ringle, C. M., & Sarstedt, M. (2015). A new criterion for assessing discriminant validity in variance-based structural equation modeling. Journal of the Academy of Marketing Science, 43(1), 115–135. https://doi.org/10.1007/s11747-014-0403-8
- Hustad, E., & Olsen, D. H. (2021). Creating a sustainable digital infrastructure: The role of service-oriented architecture. Procedia Computer Science, 181(2019), 597–604. https://doi.org/10.1016/j.procs.2021.01.210
- Jha, A. K., Agi, M. A. N., & Ngai, E. W. T. (2020). A note on big data analytics capability development in supply chain. Decision Support Systems, 138(August), 113382. https://doi.org/10.1016/j.dss.2020.113382
- Joglekar, N., Anderson, E. G., Lee, K., Parker, G., Settanni, E., & Srai, J. S. (2022). Configuration of digital and physical infrastructure platforms: Private and public perspectives. Production and Operations Management, March, 1–14. https://doi.org/10.1111/poms.13865
- Klotins, E., & Peretz-Andersson, E. (2022). The unified perspective of digital transformation and continuous software engineering. Proceedings - 5th International Workshop on Software-Intensive Business: Towards Sustainable Software Business, IWSiB 2022, 75–82. https://doi.org/10.1145/3524614.3528626
- Kock, N. (2015). Common method bias in PLS- SEM: A full collinearity assessment approach. International Journal of E- Collaboration, 11(4), 1–10. https://doi.org/10.4018/ijec.2015100101
- Kraus, S., Kallmuenzer, A., Kanbach, D. K., Krysta, P. M., & Steinhoff, M. M. (2022). An integrative framework for business model innovation in the tourism industry旅游业商 业模å¼åˆ›æ–°çš„ç»¼åˆæ¡†æž¶. The Service Industries Journal, 1–23. https://doi.org/10.1080/02642069.2022.2127690
- Kubina, M., Varmus, M., & Kubinova, I. (2015). Use of Big Data for Competitive Advantage of Company. Procedia Economics and Finance, 26(15), 561–565. https://doi.org/10.1016/s2212-5671(15)00955-7
- Lerman, L. V., Benitez, G. B., Müller, J. M., de Sousa, P. R., & Frank, A. G. (2022). Smart green supply chain management: a configurational approach to enhance green performance through digital transformation. Supply Chain Management: An International Journal, 27(7), 147–176. https://doi.org/10.1108/scm-02-2022-0059
- Manyika, J., & Roxburgh, C. (2011). The great transformer: The impact of the Internet on economic growth and prosperity. McKinsey Global Institute, 1–10. https://www.mckinsey.com/industries/technology-media-and-telecommunications/our-insights/the-great-transformer
- Mao, H., Liu, S., Zhang, J., & Deng, Z. (2016). Information technology resource, knowledge management capability, and competitive advantage: The moderating role of resource commitment. International Journal of Information Management, 36(6, Part A), 1062–1074. https://doi.org/https://doi.org/10.1016/j.ijinfomgt.2016.07.001
- Marinagi, C., Trivellas, P., & Sakas, D. P. (2014). The Impact of Information Technology on the Development of Supply Chain Competitive Advantage. Procedia - Social and Behavioral Sciences, 147, 586–591. https://doi.org/10.1016/j.sbspro.2014.07.161
- Massachusetts, C., & No, C. W. P. (2002). CENTER FOR INFORMATION SYSTEMS Massachusetts Institute of Technology IT Infrastructure for Strategic Agility Peter Weill, Mani Subramani , and Marianne Broadbent. Social Science Research.
- Naomi, S., Evans, S., Monteiro, M., & Carvalho, D. (2016). Sustainable business model innovation : Exploring evidences in sustainability reporting. Procedia CIRP, 40, 659–667. https://doi.org/10.1016/j.procir.2016.01.151
- Pakistan economic survey 2021-22. (2022.). Pakistan economic survey. Economic Survey. https://www.finance.gov.pk/survey/chapter_22/Highlights.pdf
- Parida, V., Sjödin, D., & Reim, W. (2019). Reviewing literature on digitalization, business model innovation, and sustainable industry: Past achievements and future promises. Sustainability (Switzerland), 11(2). https://doi.org/10.3390/su11020391
- Podsakoff, P. M., MacKenzie, S. B., Lee, J. Y., & Podsakoff, N. P. (2003). Common Method Biases in Behavioral Research: A Critical Review of the Literature and Recommended Remedies. Journal of Applied Psychology, 88(5), 879–903. https://doi.org/10.1037/0021-9010.88.5.879
- Rachinger, M., Rauter, R., Müller, C., Vorraber, W., & Schirgi, E. (2019). Digitalization and its influence on business model innovation. Journal of Manufacturing Technology Management, 30(8), 1143–1160. https://doi.org/10.1108/JMTM-01-2018-0020
- Ramadan, M., Shuqqo, H., Qtaishat, L., Asmar, H., & Salah, B. (2020). Sustainable competitive advantage driven by big data analytics and innovation. Applied Sciences (Switzerland), 10(19). https://doi.org/10.3390/app10196784
- Rehman, N., Razaq, S., Farooq, A., Zohaib, N. M., & Nazri, M. (2020). Information technology and firm performance: mediation role of absorptive capacity and corporate entrepreneurship in manufacturing SMEs. Technology Analysis and Strategic Management, 32(9), 1049–1065. https://doi.org/10.1080/09537325.2020.1740192
- Rialti, R., Zollo, L., Ferraris, A., & Alon, I. (2019). Big data analytics capabilities and performance: Evidence from a moderated multi-mediation model. Technological Forecasting and Social Change, 149(December). https://doi.org/10.1016/j.techfore.2019.119781
- Saeidi, P., Saeidi, S. P., Sofian, S., Saeidi, S. P., Nilashi, M., & Mardani, A. (2019). The impact of enterprise risk management on competitive advantage by moderating role of information technology. Computer Standards and Interfaces, 63(November), 67– 82. https://doi.org/10.1016/j.csi.2018.11.009
- Sarstedt, M., Hair, J. F., Cheah, J. H., Becker, J. M., & Ringle, C. M. (2019). How to specify, estimate, and validate higher-order constructs in PLS-SEM. Australasian Marketing Journal, 27(3), 197–211. https://doi.org/10.1016/j.ausmj.2019.05.003
- Saunders, M., Lewis, P., & Thornhill, A. (2007). Research Methods for Business Students. In Pearson. https://www.researchgate.net/publication/330760964_Research_Methods_for_Business_Students_Chapter_4_Understanding_research_philosophy_and_approaches_to_theory_development
- Shah, T. R. (2022). Technology in Society Can big data analytics help organisations achieve sustainable competitive advantage ? A developmental enquiry. Technology in Society, 68(October 2021), 101801. https://doi.org/10.1016/j.techsoc.2021.101801
- Shamim, S., Zeng, J., Shariq, S. M., & Khan, Z. (2019). Role of big data management in enhancing big data decision-making capability and quality among Chinese firms: A dynamic capabilities view. Information and Management, 56(6), 1–12. https://doi.org/10.1016/j.im.2018.12.003
- Sigalas, C. (2015). Competitive advantage: the known unknown concept. Management Decision, 53(9), 2004–2016. https://doi.org/10.1108/MD-05-2015-0185
- Singh, S. K., & Del Giudice, M. (2019). Big data analytics, dynamic capabilities and firm performance. Management Decision, 57(8), 1729–1733. https://doi.org/10.1108/MD-08-2019-020
- Spieth, P., Roeth, T., & Meissner, S. (2019). Reinventing a business model in industrial networks: Implications for customers’ brand perceptions. Industrial Marketing Management, 83(April), 275–287. https://doi.org/10.1016/j.indmarman.2019.04.013
- Tabesh, P., Mousavidin, E., & Hasani, S. (2019). Implementing big data strategies : Business Horizons.
- Toufaily, E., Zalan, T., & Dhaou, S. Ben. (2021). A framework of blockchain technology adoption: An investigation of challenges and expected value. Information & Management, 58(3), 103444. https://doi.org/https://doi.org/10.1016/j.im.2021.103444
- Veile, J. W., Schmidt, M.-C., & Voigt, K.-I. (2022). Toward a new era of cooperation: How industrial digital platforms transform business models in Industry 4.0. Journal of Business Research, 143, 387–405. https://doi.org/https://doi.org/10.1016/j.jbusres.2021.11.062
- Wamba, S. F., & Ren, S. J. (2017). Big data analytics and firm performance : effects of dynamic capabilities.
- Wiener, M., Saunders, C., & Marabelli, M. (2020). framework. https://doi.org/10.1177/0268396219896811
- Zhong, R. Y., Newman, S. T., Huang, G. Q., & Lan, S. (2016). Computers & Industrial Engineering Big Data for supply chain management in the service and manufacturing sectors : Challenges, opportunities, and future perspectives. Computers & Industrial Engineering, 101, 572–591. https://doi.org/10.1016/j.cie.2016.07.013
- Abeysekara, N., Wang, H., & Kuruppuarachchi, D. (2019). Effect of supply-chain resilience on firm performance and competitive advantage. Business Process Management Journal, 25(7), 1673–1695. //doi.org/10.1108/bpmj-09-2018-0241
- Al-Darras, O. M. A., & Tanova, C. (2022). From Big Data Analytics to Organizational Agility: What Is the Mechanism? SAGE Open, 12(2). https://doi.org/10.1177/21582440221106170
- Alharahsheh, H. H., & Pius, A. (2020). A Review of key paradigms: positivism VS interpretivism. Global Academic Journal of Humanities and Social Sciences, 2(3), 39–43. https://www.researchgate.net/publication/338244145
- Ambrosini, V., & Bowman, C. (2009). What are dynamic capabilities and are they a useful construct in strategic management? International Journal of Management Reviews, 11(1), 29–49. https://doi.org/10.1111/j.1468-2370.2008.00251.x
- Anwar, M. (2018). Business model innovation and SMEs performance-Does competitive advantage mediate? International Journal of Innovation Management, 22(7), 1–31. https://doi.org/10.1142/S1363919618500573
- Åström, J., Reim, W., & Parida, V. (2022). Value creation and value capture for AI business model innovation: a three-phase process framework. Review of Managerial Science, 16(7), 2111–2133. https://doi.org/10.1007/s11846-022-00521-z
- Awan, U., Bhatti, S. H., Shamim, S., Khan, Z., Akhtar, P., & Balta, M. E. (2022). The Role of Big Data Analytics in Manufacturing Agility and Performance: Moderation– Mediation Analysis of Organizational Creativity and of the Involvement of Customers as Data Analysts. British Journal of Management, 33(3), 1200–1220. https://doi.org/10.1111/1467-8551.12549
- Azeem, M., Ahmed, M., Haider, S., & Sajjad, M. (2021). Expanding competitive advantage through organizational culture, knowledge sharing and organizational innovation. Technology in Society, 66(June), 101635. https://doi.org/10.1016/j.techsoc.2021.101635
- Barlette, Y., & Baillette, P. (2022). Big data analytics in turbulent contexts: towards organizational change for enhanced agility. Production Planning and Control, 33(2–3), 105–122. https://doi.org/10.1080/09537287.2020.1810755
- Bocken, N. M. P., Short, S. W., Rana, P., & Evans, S. (2014). A literature and practice review to develop sustainable business model archetypes. Journal of Cleaner Production, 65, 42–56. https://doi.org/10.1016/j.jclepro.2013.11.039
- Bouwman, H., Nikou, S., & de Reuver, M. (2019). Digitalization, business models, and SMEs: How do business model innovation practices improve performance of digitalizing SMEs? Telecommunications Policy, 43(9), 101828. https://doi.org/10.1016/j.telpol.2019.101828
- Cappa, F., Oriani, R., Peruffo, E., & McCarthy, I. (2021). Big Data for Creating and Capturing Value in the Digitalized Environment: Unpacking the Effects of Volume, Variety, and Veracity on Firm Performance*. Journal of Product Innovation Management, 38(1), 49– 67. https://doi.org/10.1111/jpim.12545
- Chen, D. Q., Preston, D. S., & Swink, M. (2015). How the use of big data analytics affects value creation in supply chain management. Journal of Management Information Systems, 32(4), 4–39. https://doi.org/10.1080/07421222.2015.1138364
- Ciampi, F., Demi, S., Magrini, A., Marzi, G., & Papa, A. (2021a). Exploring the impact of big data analytics capabilities on business model innovation : The mediating role of entrepreneurial orientation. Journal of Business Research, 123(June 2020), 1–13. https://doi.org/10.1016/j.jbusres.2020.09.023
- Ciampi, F., Demi, S., Magrini, A., Marzi, G., & Papa, A. (2021b). Exploring the impact of big data analytics capabilities on business model innovation: The mediating role of entrepreneurial orientation. Journal of Business Research, 123(September 2020), 1– 13. https://doi.org/10.1016/j.jbusres.2020.09.023
- Cochran, D. S., Kinard, D., & Bi, Z. (2016). Manufacturing System Design meets Big Data Analytics for Continuous Improvement. Procedia CIRP, 50, 647–652. https://doi.org/10.1016/j.procir.2016.05.004
- Dahiya, R., Le, S., Ring, J. K., & Watson, K. (2022). Big data analytics and competitive advantage: the strategic role of firm-specific knowledge. Journal of Strategy and Management, 15(2), 175–193. https://doi.org/10.1108/JSMA-08-2020-0203
- Dubey, R., Gunasekaran, A., Childe, S. J., Blome, C., & Papadopoulos, T. (2019). Big Data and Predictive Analytics and Manufacturing Performance: Integrating Institutional Theory, Resource-Based View and Big Data Culture. British Journal of Management, 30(2), 341–361. https://doi.org/10.1111/1467-8551.12355
- Dubey, R., Gunasekaran, A., Childe, S. J., Luo, Z., Wamba, S. F., Roubaud, D., & Foropon, C. (2018). Examining the role of big data and predictive analytics on collaborative performance in context to sustainable consumption and production behaviour. Journal of Cleaner Production, 196, 1508–1521. https://doi.org/10.1016/j.jclepro.2018.06.097
- Garzella, S., Fiorentino, R., Caputo, A., & Lardo, A. (2021). Business model innovation in SMEs: the role of boundaries in the digital era. Technology Analysis and Strategic Management, 33(1), 31–43. https://doi.org/10.1080/09537325.2020.1787374
- Geissdoerfer, M., Vladimirova, D., & Evans, S. (2018). Sustainable business model innovation : A review. Journal of Cleaner Production, 198, 401–416. https://doi.org/10.1016/j.jclepro.2018.06.240
- Ghasemaghaei, M., & Calic, G. (2019). Does big data enhance firm innovation competency? The mediating role of data-driven insights. Journal of Business Research, 104(July), 69–84. https://doi.org/10.1016/j.jbusres.2019.07.006
- Grover, V., Chiang, R. H. L., Liang, T. P., & Zhang, D. (2018). Creating Strategic Business Value from Big Data Analytics: A Research Framework. Journal of Management Information Systems, 35(2), 388–423. https://doi.org/10.1080/07421222.2018.1451951
- Gupta, S., Drave, V. A., Dwivedi, Y. K., Baabdullah, A. M., & Ismagilova, E. (2020). Achieving superior organizational performance via big data predictive analytics: A dynamic capability view. Industrial Marketing Management, 90, 581– 592. https://doi.org/10.1016/j.indmarman.2019.11.009
- Gupta, S., Justy, T., Kamboj, S., Kumar, A., & Kristoffersen, E. (2021). Big data and firm marketing performance: Findings from knowledge-based view. Technological Forecasting and Social Change, 171(1). https://doi.org/10.1016/j.techfore.2021.120986
- Hair, J. F., Risher, J. J., Sarstedt, M., & Ringle, C. M. (2019). When to use and how to report the results of PLS-SEM. European Business Review, 31(1), 2–24. https://doi.org/10.1108/ebr-11-2018-0203
- Hair, J. F. (2021). Reflections on SEM: An Introspective, Idiosyncratic Journey to Composite-Based Structural Equation Modeling. The DATA BASE for Advances in Information Systems, 52(SI), 101–113. http://dx.doi.org/10.1145/3505639.3505646
- Henseler, J., Ringle, C. M., & Sarstedt, M. (2015). A new criterion for assessing discriminant validity in variance-based structural equation modeling. Journal of the Academy of Marketing Science, 43(1), 115–135. https://doi.org/10.1007/s11747-014-0403-8
- Hustad, E., & Olsen, D. H. (2021). Creating a sustainable digital infrastructure: The role of service-oriented architecture. Procedia Computer Science, 181(2019), 597–604. https://doi.org/10.1016/j.procs.2021.01.210
- Jha, A. K., Agi, M. A. N., & Ngai, E. W. T. (2020). A note on big data analytics capability development in supply chain. Decision Support Systems, 138(August), 113382. https://doi.org/10.1016/j.dss.2020.113382
- Joglekar, N., Anderson, E. G., Lee, K., Parker, G., Settanni, E., & Srai, J. S. (2022). Configuration of digital and physical infrastructure platforms: Private and public perspectives. Production and Operations Management, March, 1–14. https://doi.org/10.1111/poms.13865
- Klotins, E., & Peretz-Andersson, E. (2022). The unified perspective of digital transformation and continuous software engineering. Proceedings - 5th International Workshop on Software-Intensive Business: Towards Sustainable Software Business, IWSiB 2022, 75–82. https://doi.org/10.1145/3524614.3528626
- Kock, N. (2015). Common method bias in PLS- SEM: A full collinearity assessment approach. International Journal of E- Collaboration, 11(4), 1–10. https://doi.org/10.4018/ijec.2015100101
- Kraus, S., Kallmuenzer, A., Kanbach, D. K., Krysta, P. M., & Steinhoff, M. M. (2022). An integrative framework for business model innovation in the tourism industry旅游业商 业模å¼åˆ›æ–°çš„ç»¼åˆæ¡†æž¶. The Service Industries Journal, 1–23. https://doi.org/10.1080/02642069.2022.2127690
- Kubina, M., Varmus, M., & Kubinova, I. (2015). Use of Big Data for Competitive Advantage of Company. Procedia Economics and Finance, 26(15), 561–565. https://doi.org/10.1016/s2212-5671(15)00955-7
- Lerman, L. V., Benitez, G. B., Müller, J. M., de Sousa, P. R., & Frank, A. G. (2022). Smart green supply chain management: a configurational approach to enhance green performance through digital transformation. Supply Chain Management: An International Journal, 27(7), 147–176. https://doi.org/10.1108/scm-02-2022-0059
- Manyika, J., & Roxburgh, C. (2011). The great transformer: The impact of the Internet on economic growth and prosperity. McKinsey Global Institute, 1–10. https://www.mckinsey.com/industries/technology-media-and-telecommunications/our-insights/the-great-transformer
- Mao, H., Liu, S., Zhang, J., & Deng, Z. (2016). Information technology resource, knowledge management capability, and competitive advantage: The moderating role of resource commitment. International Journal of Information Management, 36(6, Part A), 1062–1074. https://doi.org/https://doi.org/10.1016/j.ijinfomgt.2016.07.001
- Marinagi, C., Trivellas, P., & Sakas, D. P. (2014). The Impact of Information Technology on the Development of Supply Chain Competitive Advantage. Procedia - Social and Behavioral Sciences, 147, 586–591. https://doi.org/10.1016/j.sbspro.2014.07.161
- Massachusetts, C., & No, C. W. P. (2002). CENTER FOR INFORMATION SYSTEMS Massachusetts Institute of Technology IT Infrastructure for Strategic Agility Peter Weill, Mani Subramani , and Marianne Broadbent. Social Science Research.
- Naomi, S., Evans, S., Monteiro, M., & Carvalho, D. (2016). Sustainable business model innovation : Exploring evidences in sustainability reporting. Procedia CIRP, 40, 659–667. https://doi.org/10.1016/j.procir.2016.01.151
- Pakistan economic survey 2021-22. (2022.). Pakistan economic survey. Economic Survey. https://www.finance.gov.pk/survey/chapter_22/Highlights.pdf
- Parida, V., Sjödin, D., & Reim, W. (2019). Reviewing literature on digitalization, business model innovation, and sustainable industry: Past achievements and future promises. Sustainability (Switzerland), 11(2). https://doi.org/10.3390/su11020391
- Podsakoff, P. M., MacKenzie, S. B., Lee, J. Y., & Podsakoff, N. P. (2003). Common Method Biases in Behavioral Research: A Critical Review of the Literature and Recommended Remedies. Journal of Applied Psychology, 88(5), 879–903. https://doi.org/10.1037/0021-9010.88.5.879
- Rachinger, M., Rauter, R., Müller, C., Vorraber, W., & Schirgi, E. (2019). Digitalization and its influence on business model innovation. Journal of Manufacturing Technology Management, 30(8), 1143–1160. https://doi.org/10.1108/JMTM-01-2018-0020
- Ramadan, M., Shuqqo, H., Qtaishat, L., Asmar, H., & Salah, B. (2020). Sustainable competitive advantage driven by big data analytics and innovation. Applied Sciences (Switzerland), 10(19). https://doi.org/10.3390/app10196784
- Rehman, N., Razaq, S., Farooq, A., Zohaib, N. M., & Nazri, M. (2020). Information technology and firm performance: mediation role of absorptive capacity and corporate entrepreneurship in manufacturing SMEs. Technology Analysis and Strategic Management, 32(9), 1049–1065. https://doi.org/10.1080/09537325.2020.1740192
- Rialti, R., Zollo, L., Ferraris, A., & Alon, I. (2019). Big data analytics capabilities and performance: Evidence from a moderated multi-mediation model. Technological Forecasting and Social Change, 149(December). https://doi.org/10.1016/j.techfore.2019.119781
- Saeidi, P., Saeidi, S. P., Sofian, S., Saeidi, S. P., Nilashi, M., & Mardani, A. (2019). The impact of enterprise risk management on competitive advantage by moderating role of information technology. Computer Standards and Interfaces, 63(November), 67– 82. https://doi.org/10.1016/j.csi.2018.11.009
- Sarstedt, M., Hair, J. F., Cheah, J. H., Becker, J. M., & Ringle, C. M. (2019). How to specify, estimate, and validate higher-order constructs in PLS-SEM. Australasian Marketing Journal, 27(3), 197–211. https://doi.org/10.1016/j.ausmj.2019.05.003
- Saunders, M., Lewis, P., & Thornhill, A. (2007). Research Methods for Business Students. In Pearson. https://www.researchgate.net/publication/330760964_Research_Methods_for_Business_Students_Chapter_4_Understanding_research_philosophy_and_approaches_to_theory_development
- Shah, T. R. (2022). Technology in Society Can big data analytics help organisations achieve sustainable competitive advantage ? A developmental enquiry. Technology in Society, 68(October 2021), 101801. https://doi.org/10.1016/j.techsoc.2021.101801
- Shamim, S., Zeng, J., Shariq, S. M., & Khan, Z. (2019). Role of big data management in enhancing big data decision-making capability and quality among Chinese firms: A dynamic capabilities view. Information and Management, 56(6), 1–12. https://doi.org/10.1016/j.im.2018.12.003
- Sigalas, C. (2015). Competitive advantage: the known unknown concept. Management Decision, 53(9), 2004–2016. https://doi.org/10.1108/MD-05-2015-0185
- Singh, S. K., & Del Giudice, M. (2019). Big data analytics, dynamic capabilities and firm performance. Management Decision, 57(8), 1729–1733. https://doi.org/10.1108/MD-08-2019-020
- Spieth, P., Roeth, T., & Meissner, S. (2019). Reinventing a business model in industrial networks: Implications for customers’ brand perceptions. Industrial Marketing Management, 83(April), 275–287. https://doi.org/10.1016/j.indmarman.2019.04.013
- Tabesh, P., Mousavidin, E., & Hasani, S. (2019). Implementing big data strategies : Business Horizons.
- Toufaily, E., Zalan, T., & Dhaou, S. Ben. (2021). A framework of blockchain technology adoption: An investigation of challenges and expected value. Information & Management, 58(3), 103444. https://doi.org/https://doi.org/10.1016/j.im.2021.103444
- Veile, J. W., Schmidt, M.-C., & Voigt, K.-I. (2022). Toward a new era of cooperation: How industrial digital platforms transform business models in Industry 4.0. Journal of Business Research, 143, 387–405. https://doi.org/https://doi.org/10.1016/j.jbusres.2021.11.062
- Wamba, S. F., & Ren, S. J. (2017). Big data analytics and firm performance : effects of dynamic capabilities.
- Wiener, M., Saunders, C., & Marabelli, M. (2020). framework. https://doi.org/10.1177/0268396219896811
- Zhong, R. Y., Newman, S. T., Huang, G. Q., & Lan, S. (2016). Computers & Industrial Engineering Big Data for supply chain management in the service and manufacturing sectors : Challenges, opportunities, and future perspectives. Computers & Industrial Engineering, 101, 572–591. https://doi.org/10.1016/j.cie.2016.07.013
Cite this article
-
APA : Rizvi, S. A. A., Ali, M., & Raza, H. (2023). The Role of Big Data Analytics Capability to Achieve Competitive Advantage with the Mediation of Business Model Innovation: A Dynamic Capability View. Global Management Sciences Review, VIII(I), 34-53. https://doi.org/10.31703/gmsr.2023(VIII-I).03
-
CHICAGO : Rizvi, Syed Aamir Alam, Mohsin Ali, and Hasan Raza. 2023. "The Role of Big Data Analytics Capability to Achieve Competitive Advantage with the Mediation of Business Model Innovation: A Dynamic Capability View." Global Management Sciences Review, VIII (I): 34-53 doi: 10.31703/gmsr.2023(VIII-I).03
-
HARVARD : RIZVI, S. A. A., ALI, M. & RAZA, H. 2023. The Role of Big Data Analytics Capability to Achieve Competitive Advantage with the Mediation of Business Model Innovation: A Dynamic Capability View. Global Management Sciences Review, VIII, 34-53.
-
MHRA : Rizvi, Syed Aamir Alam, Mohsin Ali, and Hasan Raza. 2023. "The Role of Big Data Analytics Capability to Achieve Competitive Advantage with the Mediation of Business Model Innovation: A Dynamic Capability View." Global Management Sciences Review, VIII: 34-53
-
MLA : Rizvi, Syed Aamir Alam, Mohsin Ali, and Hasan Raza. "The Role of Big Data Analytics Capability to Achieve Competitive Advantage with the Mediation of Business Model Innovation: A Dynamic Capability View." Global Management Sciences Review, VIII.I (2023): 34-53 Print.
-
OXFORD : Rizvi, Syed Aamir Alam, Ali, Mohsin, and Raza, Hasan (2023), "The Role of Big Data Analytics Capability to Achieve Competitive Advantage with the Mediation of Business Model Innovation: A Dynamic Capability View", Global Management Sciences Review, VIII (I), 34-53
-
TURABIAN : Rizvi, Syed Aamir Alam, Mohsin Ali, and Hasan Raza. "The Role of Big Data Analytics Capability to Achieve Competitive Advantage with the Mediation of Business Model Innovation: A Dynamic Capability View." Global Management Sciences Review VIII, no. I (2023): 34-53. https://doi.org/10.31703/gmsr.2023(VIII-I).03